👋 Guest author Lindsay Jenkins studies bioethics, cognitive science and classics at Georgetown University.
The Map of Science and its millions of global research publications can be daunting at first - believe us, we know. (The title of this post was almost “I've come around - the Map of Science is actually pretty cool.”) We find that tinkering with the Map is the best way to get over the initial intimidation. In this post, I'll share how I used the Map to examine how AI is being used in reproductive healthcare, a topic that especially interests me as a student of bioethics.
Upon opening the Map, I'm met with a array of clusters, or groups of research articles that cite each other often. My goal here is to narrow down what looks like a convoluted neural network (as in encephalic, rather than CNN) to only artificial intelligence as it is used in reproductive healthcare. To accomplish this, I started by selecting the subject filters for “artificial intelligence” and “gynecology,” toggling the “or/and” button to “and.”
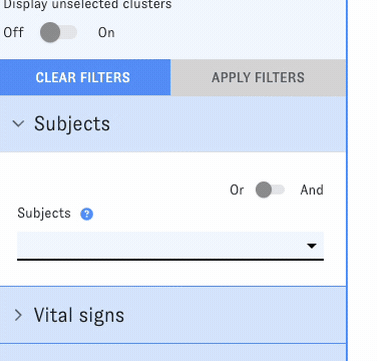
I then repeated this process substituting “obstetrics” with “gynecology.” This gave me two sets of clusters: one for AI and gynecology, one for AI and obstetrics. Then, I used the Map's list view to skim each set, pulling out clusters with key concepts that seemed to me to be directly related to gynecology and obstetrics.
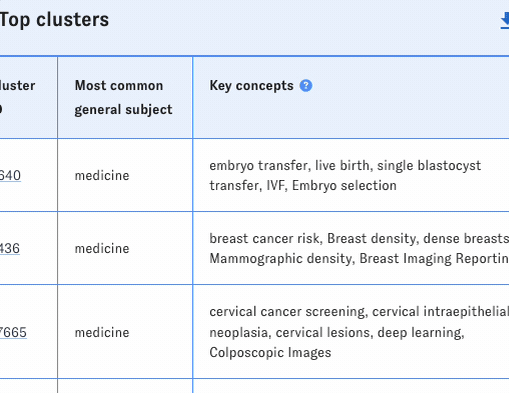
My final list included eight clusters: 39966, 19829, 6640, 3436, 27665, 55792, 26674, and 53534. I reset the Map and focused on these eight clusters using the "Cluster ID" input (located under "Advanced filters" in the filter pane).
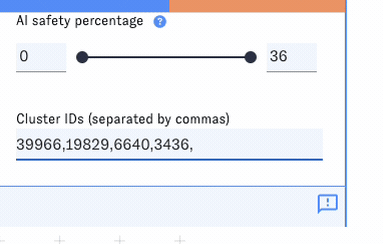
According to the Map's list view, AI relevance for these clusters ranged from 11.5% (19829) to 35.4% (27665), with key concepts including cervical cancer, deep learning, breast ultrasound imaging, mammography, IVF, preterm labor prediction, fetal lung maturation, and neonatal mortality, among others.
Switching back to map view, we can see that the eight clusters triangulate themselves, with 53534 and 5572 at one point (fetal and neonatal medicine); 39966, 6640, 26674 at another (IVF, labor, and birth); and 27665, 3436, and 19829 at another (cervical and breast cancer screening). Clusters that are closer together in the Map have more intercluster citation links, meaning that within each of these three groups, there is a relatively high amount of citations from publications in one cluster to publications in the others.
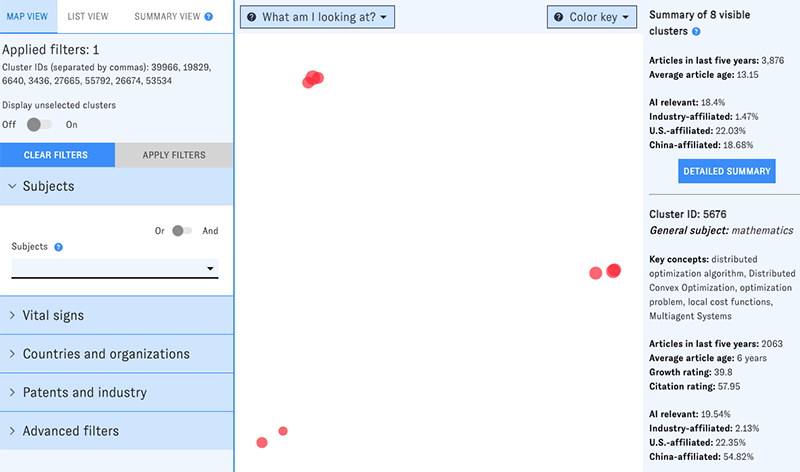
Finally, I switched to summary view to generate an overall synopsis of the eight clusters. Some highlights from the analysis:
- Of the eight selected clusters, 19829 (computer vision and breast cancer screening) has a notably high growth rating (67th percentile), while 6640 (IVF and embryonic medicine) is notably large overall (1,174 recent articles).
- Across the eight clusters, about 19% of articles are AI-related. 12%, or nearly two-thirds of these AI-related articles, are related to computer vision in particular. This may suggest that computer vision is an especially important AI discipline for obstetrics and gynecology, as compared to other AI subfields (such as robotics or natural language processing).
- Across the eight clusters, the United States was the most common author and funder country. Papers from UK authors ranked first in yearly citations (3.43). Across both top author and top funding countries, China came in a close second for articles published.
- Major funding organizations included Harvard University, Brigham and Women’s Hospital, and Massachusetts General Hospital.
Generally, these results reinforce how computer vision has promising applications for diagnostic purposes, both generally and in women's reproductive health in particular. For example, CNNs and deep learning algorithms can recognize uterine contractions with electrohysterography and predict preterm births, induction success, and IVF embryo ploidy and viability for implantation as well as classify cervical cancers and automate breast ultrasounds. The applicability of these algorithms are transferable to a multitude of other purposes such as endoscopic polyp identification, skin cancer detection, and x-ray image reading, as well.
The results may also point to areas where commitment to OB/GYN AI innovation could be increased. My quick search turned up multiple clusters on breast cancer screening with very high proportions of AI-relevant articles, but (for example) only one cluster on cervical cancer screening, with a lower AI proportion. This might suggest that even within OB/GYN, the same kinds of algorithmic tools (for example, the same type of computer vision algorithm) may be used for some types of diagnoses more than others.
This was just a brief dive into a cluster-case study of biotechnology in women’s health, but the Map can be applied to any subject or combination of subjects found in the Map's subject filter. Check out AI + law and economics here if you’re interested in copyright law or deontic logic, or run this query for alpaca fiber, dairy cows, and wild blueberry fields under an AI + animal science combination.
As always, we're glad to help you explore the Map - visit our support hub to contact us, book live support with an ETO staff member or access the latest documentation for our tools and data. 🤖