Attention Substack users! ETO blog posts are also available on Substack.
AI research is booming. Keeping up with thousands of new articles a month can seem like an impossible task - but for those who want to understand this technology, there's no substitute for following the research. That's because the field of AI has a remarkably open research culture, with scientists and developers sharing new results daily in preprint servers, journals and a dizzying range of conferences. Though some aspects of this culture may be beginning to shift, understanding the research landscape will remain a critical way to understand modern AI and where it's headed.
Still, the sheer volume can make it nearly impossible to figure out where to focus. Luckily, many of our ETO tools are designed to help. In another blog post, we survey global AI research at a high level using ETO's Research Almanac. In this one, we'll share one of our favorite strategies for using the Map of Science to hone in on critical pockets of AI research around the world.
Check out the companion post to explore high-level trends in AI research around the world: The state of global AI research
The Map of Science organizes hundreds of millions of global research publications into research clusters, which are groups of articles that cite each other often - typically because they share other things in common, such as topics, concepts, or languages. You can use the Map's built-in filters to narrow down to clusters that are relevant to a particular subject, or based on other characteristics, like growth rate, country, or organization.
Map of Science users often want to learn about the "top" areas of research on a particular subject. There could be many ways to define "top" research, of course, but ETO analysts often use a combination of the Map's growth and scale concepts to begin the analysis. With this approach, we look for research clusters that are both unusually large and have lots of recent articles - a rare pairing.
Let's see how this works for AI in particular. Starting from the Map's list view, I'll filter down to research clusters with a growth rating of 90 or higher (that is, clusters with a higher proportion of recently published articles than 90% or more of all clusters in the Map) and with at least 2,000 new articles in the last five years. (You could experiment with different thresholds on each filter; here, I'm choosing very high thresholds in order to identify truly exceptional clusters.)
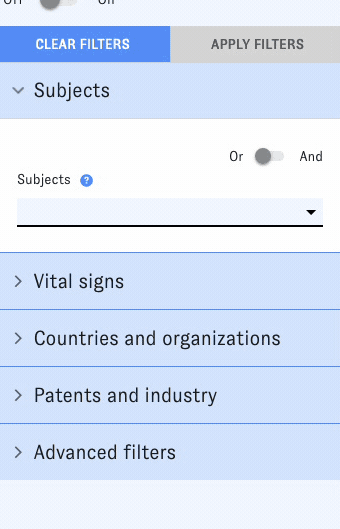
Out of nearly 86,000 clusters in the Map, only 39 clusters meet these filters as of the publication of this post - a tiny slice of the broader research landscape. Next, I'll use the subjects menu to identify the clusters with lots of AI-related research.
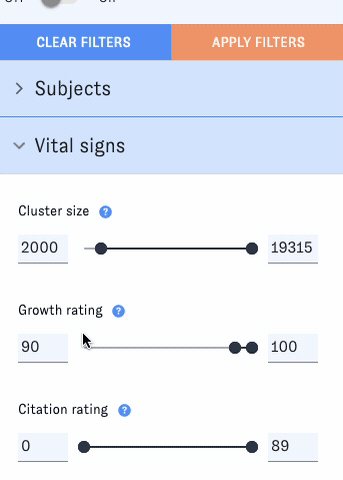
21 clusters remain. That's an interesting finding in itself: over half of the top research clusters in the Map of Science are AI-related (using our particular definition of "top").
What exactly are these clusters about? With the Map's key concepts feature and a few minutes spent browsing the twenty top AI clusters' detail views, we can start getting a rough lay of the land:
To those who follow AI research trends, these 20 top clusters form a microcosm of the field as a whole. Several critical strands of research appear to be represented:
- Research on basic AI approaches and capabilities, with a massive and quickly growing body of work on large language models (#1407) unsurprisingly leading the way. Other clusters in the top 20 focus on high-level AI approaches such as federated learning (#9948), a set of techniques for coordinating complex AI-related algorithms across many computers, and diffusion models (#7917), a type of AI model architecture often used for multimedia.
- Research on applying AI to real-world needs and problems. Some of the Map's most prominent AI clusters focus on using machine learning to diagnose plant diseases (#5167), another on helping autonomous vehicles detect nearby objects (#25074), another on using AI to monitor lithium-ion batteries (#21023), another on AI models that can generate and understand computer code (#26579), and so on - the spectrum of emerging AI applications is as broad as science itself.
- Research on AI safety and security - in a nutshell, identifying the many surprises and downsides modern AI continues to reveal, and working to mitigate them. Several of the top AI clusters in our results tackle well-known challenges in this domain, including gender bias in large language models (#44973), manipulation of facial recognition systems (#40959), and AI models inadvertently leaking potentially confidential training data to attackers (#40161)
- Research on society's response to AI - for example, strategies for building AI literacy as part of K-12 education (#27139) or integrating AI into business processes (#74631).
As next steps, you might use the Map to identify key countries and organizations in these different areas of AI research - or run a similar "top clusters" analysis for subjects other than AI. How about chemistry, radiology, or robotics?
As always, we're glad to help you get the most out of the Map of Science and our other resources. Visit our support hub to contact us, book live support with an ETO staff member or access the latest documentation for our tools and data. 🤖